- HOME
- Research
- Researcher's Profile
- Yusuke MUKUTA
Researcher's Profile
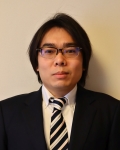
- Lecturer
- Yusuke MUKUTA
- Machine Intelligence
Biography
March 2018 | PH. D of Information Science and Technology, the University of Tokyo (UTokyo) |
---|---|
April 2018 | Assistant Professor, Department of Mechano-Informatics, Graduate School of Information Science and Technology, UTokyo |
October 2018 | Visiting Researcher, RIKEN AIP |
May 2020 | Lecturer, RCAST, UTokyo |
Research Interests
Machine learning and feature extraction tasks are very fundamental for the computer to recognize the real world. Our research objective is to propose the efficient algorithms and their analysis based on some mathematical viewpoints for these tasks.
Below are the selected research topics:
(1) Probabilistic models for calculating the causality measure
Causality measure is the measure to estimate the causal effect from one time series to the other. We proposed a novel probabilistic models that is equivalent to the existing method and also proposed its Bayesian extension such that we can estimate the measure using fewer samples. Furthermore, we extended the model such that we can estimate the measure from the samples with missing values by applying semi-supervised learning technique.
(2) Feature extraction from the viewpoint of kernel approximation
We proposed a novel methods for local feature extraction, feature coding and feature pooling tasks used for image recognition. Proposed method enabled us to recognize well with lower feature dimension and analyze the performance from the viewpoint of kernel.
(3) Feature extraction based on invariance
The invariance information about what sort of transformations preserve the contents of input data is important for constructing feature function. We combined the group representation theory that can handle the invariance mathematically with feature coding methods and proposed the invariant extensions of existing coding methods. We also work on the research to combine the group representation theory with Deep Neural Networks to construct the invariant feature extraction networks.
Keywords
Image Recognition, Machine Learning, Feature Extraction
Educational Systems
- Department of Advanced Interdisciplinary Studies, Graduate school of Engineering