- HOME
- Research
- Researcher's Profile
- Hiroki UEDA
Researcher's Profile
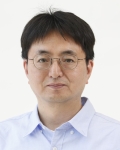
- Project Lecturer
- Hiroki UEDA
- Advanced Data Science
Biography
August 2000 | BSc,Mathematics, University of Victoria |
---|---|
October 2000 | Inter Quest Co.,Ltd. |
August 2001 | Softwave Corp. |
December 2002 | Metropolitan Computer Engineer Association |
March 2003 | M.S, Graduate School of Engineering, Kanazawa Institute of Technology |
September 2006 | Resercher,Japan Biological Informatics Consortium |
April 2010 | Intec Inc. |
September 2013 | PhD, School of Engineering, The University of Tokyo (UTokyo) |
April 2015 | Resercher, Fujitsu Limited |
March 2018 | Lecturer, RCAST, UTokyo |
Research Interests
With the development of sequencing technology, electronic data yields in biology have been steadily increasing, and it is already a challenging task to process large volumes of data in a conventional method. In addition, in order to extract knowledge from multi modal big data, (ex. Multi-omics data) it is necessary to incorporate the latest Data Science technology, such as cloud computing and machine learning. Research topics include following.
1. Epitranscriptome analysis Epitranscriptome is transcriptomics with biochemical modifications of RNA. In previous studies, we have developed a bioinformatics method to comprehensively detect inosine-modified sites in the transcriptome at the base level.
2. Cancer genomics With using next generation sequencer (NGS), it became feasible to detect cancer somatic mutations comprehensively, and NGS is now used as clinical applications, in additions to a research use. Because the allelic fraction of a mutation depends on the tumor purity, local copy number and clonality, it is sometime difficult to call somatic mutation with high accuracy with different specimen. In previous studies, we developed algorithms to calculate somatic mutations, copy number mutations and tumor rates in cancer cells even under noisy low tumor purity conditions.
3. Bioinformatics data analysis using Data Science In order to find the biological knowledge from biological big data, it is necessary to aggregate data on a cloud and perform distributed processing. We are developing cloud based NGS analysis pipeline using Hadoop / Spark , popular cloud computing framework, and deep learning library.
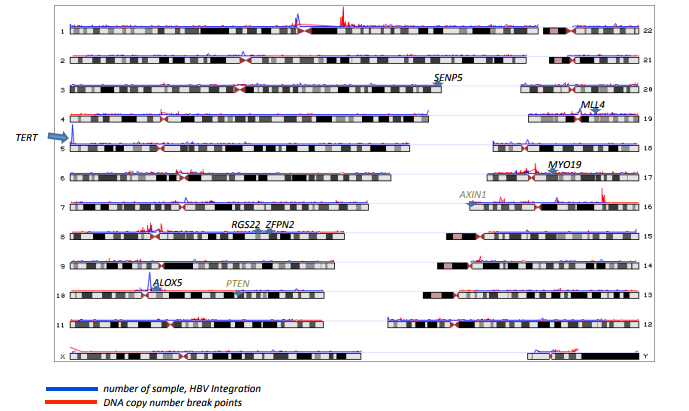
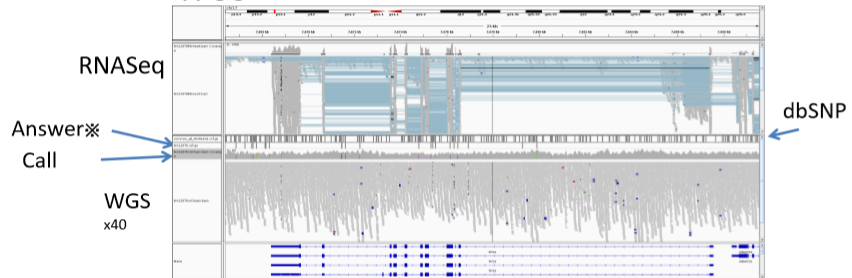
Keywords
Biological Data Science, Bioinformatics, Cancer Genomics, Machine Learning
Educational Systems
- Department of Advanced Interdisciplinary Studies, Graduate school of Engineering